The beauty of randomness
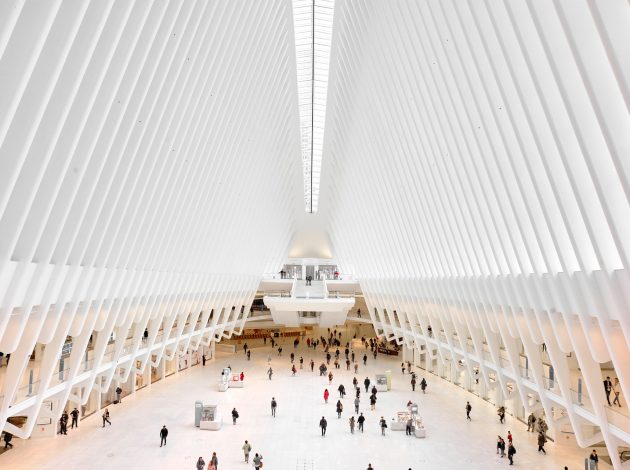
Jonathan Athow explains how the principle of randomness lies at the heart of statistics.
The idea of randomness is not one that appeals to many people. It implies a lack of control and smacks of the ‘slings and arrows of outrageous fortune’. For many people, and in many situations, randomness is bad.
Statisticians view it differently. There is a beauty or magic to randomness that unlocks our understanding of the world. Without randomness, we would know a lot less than we do now.
Opinion polling
Let me illustrate the point by looking at opinion polling during the 1936 US Presidential Election. During the election campaign, a prominent magazine – the Literary Digest – ran an opinion poll with over 2 million respondents. It suggested the Republican candidate would win by a handsome margin.
George Gallup (who went on to create the Gallup polling organisation) also ran a poll, but had just 50,000 replies. He forecast that the Democratic candidate would win. Despite having a little over 2 per cent of the respondents of the Literary Digest, his forecast was much more accurate. When the Democrats won, Gallup’s poll was lauded and it set in train the interest in opinion polls that we still see until this day.
One of the reasons that the Literary Digests’ poll failed was their sample was not random. For example, the respondents were drawn from its readers who were more likely to be Republican voters. On the other hand, Gallup’s poll had a more sophisticated approach to sampling.
“If we have selected you at random, we need you to fill in the survey to help the randomness do its work.”
A genuinely random sample is hugely powerful. Say I wanted to understand the answer to a simple yes / no question (for example, are you right handed?). If I sampled 1,000 people at random, I would be able to infer the position for the population at large to within an expected accuracy of around plus or minus 3 per cent. More amazingly (in my view anyway), the number of people I need to survey is 1,000 irrespective of the size of the whole population.
Reliable estimate
So if I was to survey 1,000 people at random from Iceland’s 330,000 population I would have a margin of error of 3 per cent. But if I was to draw 1,000 people randomly from China’s 1.4 billion population, I would also have the same 3 per cent margin of error. Randomness allows me to pick a small proportion of the population (just 0.00007 per cent in the case of China) and be able to make a reliable estimate for the population as a whole. I cannot offer any particular intuition here; this result falls out of the maths of dealing with random samples.
I have used examples of election polling to motivate this discussion, but the approach of random probability sampling underpins virtually all of ONS’s surveys. In turn, these surveys are used to understand issues such as employment and unemployment, household income, GDP, crime and many more.
While the mathematics of randomness is clear, the practicalities are harder. How do we get a genuinely random sample? This means, for example, properly defining the whole population and being alive to unequal response rates. There are tools and techniques for dealing with these issues, and sometimes that means we need a larger sample size than the raw numbers would suggest.
Statistical challenges
Randomness can also get over other statistical challenges. When we ask businesses or individuals to complete surveys, we know some of the answers will be estimates rather than the true figure. In statistical terms, the difference between the estimates and true value are known as ‘errors’. If those errors are random – not an unreasonable assumption – a large enough sample will give us a reliable estimate of the true value.
The principle of randomness is at the heart of statistics, and therefore vital to our understanding of the society and economy in which we live. It underpins much of our knowledge. Randomness therefore has a beauty to it. However, it is very important that people fill in our surveys: if we have selected you at random, we need you to fill in the survey to help the randomness do its work.
Jonathan Athow is Deputy National Statistician