Far from average: How COVID-19 has impacted the Average Weekly Earnings data
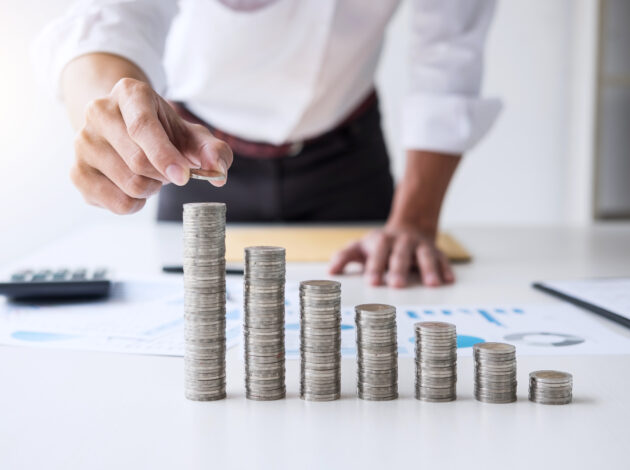
The pandemic has affected many of the figures that the ONS publishes – both in terms of ‘real world’ changes to society and the economy, and also in the picture that our standard metrics can give. Here Jonathan Athow looks at just one of these areas – our statistics on average earnings.
Interpreting average earnings data is difficult at the moment. There are temporary factors that have increased the headline growth rate in earnings above the underlying rate. To help users, we are highlighting how you might derive an underlying growth rate. I am afraid there is no simple answer, but I will take you through our thinking.
Our monthly measure of earnings is Average Weekly Earnings, and it does exactly what it says on the tin. It is simply the average (mean) of weekly earnings for all employees, and in the most recent period (May 2021) that is around £540 a week. We typically compare wages this year with wages 12 months ago to give us a measure of earnings growth. Positive earnings growth is capturing the improvement in earnings over the course of the year.
There are two reasons why headline average earnings are higher than the underlying rate at the moment. Firstly, there is something called a ‘base effect’. In spring-summer 2020, many workers were on furlough or had their hours reduced. This meant that people saw their earnings fall, pushing down weekly wages. This year, with fewer people on furlough and hours returning closer to normal, weekly wages are higher.
Thus some of the reason we have a higher growth rate this year is because some wages were falling last year. Earnings growth is capturing an improvement in earnings, but because we start from a low base that improvement is overstated. These base effects are common in statistics, but what makes them more pronounced now is the huge economic shock that the pandemic created.
There are a number of ways you can try to strip out these base effects, but no single method everyone would agree on. We have tried a couple of simple approaches.
- Firstly, we project forward earnings into spring-summer 2020 using pre-pandemic trends. We estimate what 2020 earnings would have been without the pandemic and then compare it with actual levels today.
- Secondly, we take the growth rate over the two years by comparing 2021 and 2019 earnings. We then turn that into an annual average growth rate over the two years.
Neither approach is perfect: the first requires an estimate of what would have happened without the pandemic, and the second assumes that wage growth was constant over the last two years. So we use these to generate a range fio the base effect, to which we will come back later.
Moving on from base effects, the second challenge is what we call ‘compositional effects’. As we are taking a simple average, the make-up of the employees captured in Average Weekly Earnings affects that average. During the pandemic, we saw lower-paid people at greater risk of losing their jobs. Fewer lower-paid people in the workforce increased average earnings for those who remained in work.
The analogy I like to use is height. If the shortest person in a room leaves, the average height of those remaining will rise. No-one has got taller, but the composition of the people in the room has changed, pushing up average height. In terms of average earnings, if someone paid less than the average (£540 a week) loses their job, other things equal, the average earnings will increase.
These compositional effects are always with us, and are very difficult to control for. One approach is to look at the relationship between those losing jobs and their wages and to work out what earnings would have been if these people had not lost their employment. Exactly how you do this is complex, and requires calculations using different datasets. Nonetheless, we have estimated this effect and can apply it to average earnings.
A further challenge with the compositional effect is that it is not constant over time. We are comparing the composition of employees now with a year ago, and broadly speaking the more dissimilar they are, the greater the effect. But the greatest fall in employees came early on the pandemic, so as we move further on those compositional effects are already in the base period. Other things being equal, this compositional effect should fall over time, and could even go into reverse.
The reason for the detailed explanation here is to make the point that removing base and compositional effects is not straightforward, and often there is just not one way of doing this that everyone would agree on. So any estimates of underlying growth rates are uncertain and need to be treated with caution.
So where does that leave us?
Well, this month the headline regular earnings growth rate is 6.6%. We estimate that the base effect would reduce the headline rate by between 1.8 and 3.0 percentage points based on the two methods set out above. In addition, the compositional effect we estimate at 0.4 percentage points above pre-pandemic levels. This would give an underlying rate of between 3.2% and 4.4%.
We continue to report our headline earnings statistics, but at this time feel it important to explain to users that there are different effects that mean our statistics need to be interpreted with caution.
Our calculations of an underlying rate are there to help users understand base and compositional effects, but at risk of repeating myself, there remains a lot of uncertainty about how best to control for these effects.
We continue to work on issues such as compositional effects, where there are a number of methods used internationally. Our job is not just to produce statistics, but to explain them and continue to explore ways in which they can be improved.
Jonathan Athow is Deputy National Statistician for Economic Statistics.